Abstract
Predicting the movement of crowds in a city is strategically important for traffic management, risk assessment, and public safety. In this paper, we propose the novel problem of predicting two types of flows of crowds in every region of a city based on big data, including human mobility data, weather conditions, and road network data. To have a practical solution for citywide traffic prediction, we first partition the map of a city into regions using both its road network and historical records of human mobility. Our problem is different from the predictions of each individual’s movements and each road segment’s traffic conditions, which are computationally
costly and not necessary from the perspective of public safety at a citywide scale. To model the multiple complex factors aaffecting the crowd flows, we decompose the
costly and not necessary from the perspective of public safety at a citywide scale. To model the multiple complex factors aaffecting the crowd flows, we decompose the
flows into three components: seasonal (periodic patterns), trend (changes in periodic patterns), and residual flows (instantaneous changes). The seasonal and trend models are built as intrinsic Gaussian Markov random fields, which can cope with noisy and missing data. Whereas, the residual model exploits the spatio-temporal dependence among different flows and regions, as well as the effect of weather. Experimental results on three real-world datasets show that our method is scalable and outperforms all baselines significantly in accuracy.
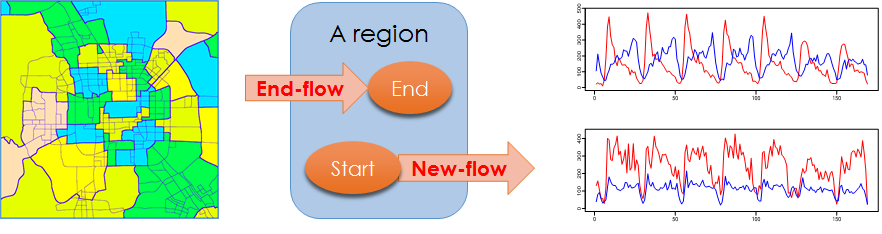